Wittawat Jitkrittum
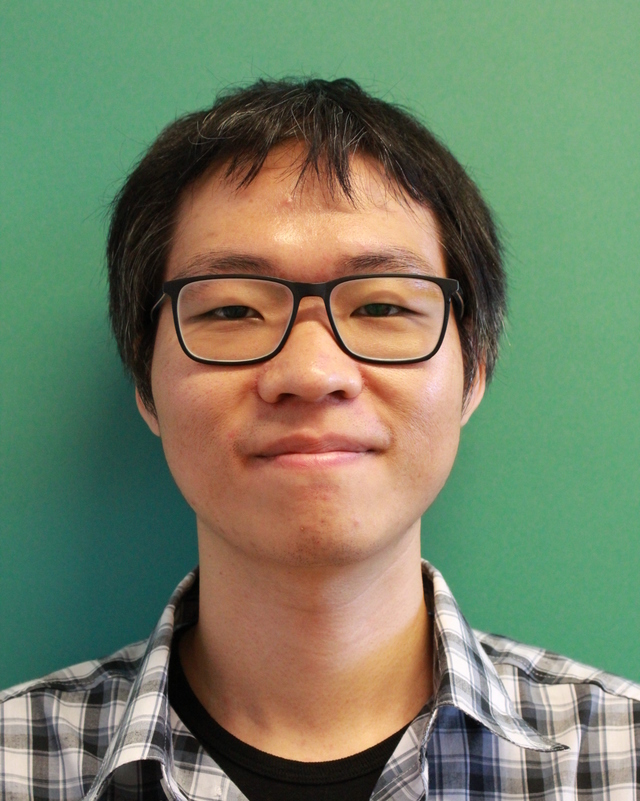
I am a research scientist at Google Research. Previously I was a postdoctoral researcher at Empirical Inference Department, Max Planck Institute for Intelligent Systems working with Bernhard Schölkopf (from 2018 to 2020). I work in the field of machine learning (intersection of computer science and statistics). My research topics include (but not limited to)
- Fast (linear runtime) non-parametric statistical tests
- Kernel-based representation of data
- Deep generative modelling of images
- Approximate Bayesian inference
Contact: Wittawat Jitkrittum (วิทวัส จิตกฤตธรรม) ( )
ArXiv CV DBLP Github Google Scholar LinkedIn Orcid ID ResearchGate Semantic Scholar Twitter
Last update: 22-Jan-24
Based on al-folio theme.
News
16 Jan 2024 |
|
11 Dec 2023 |
|